Leading the Charge
AI & ML in Modern Security Operations
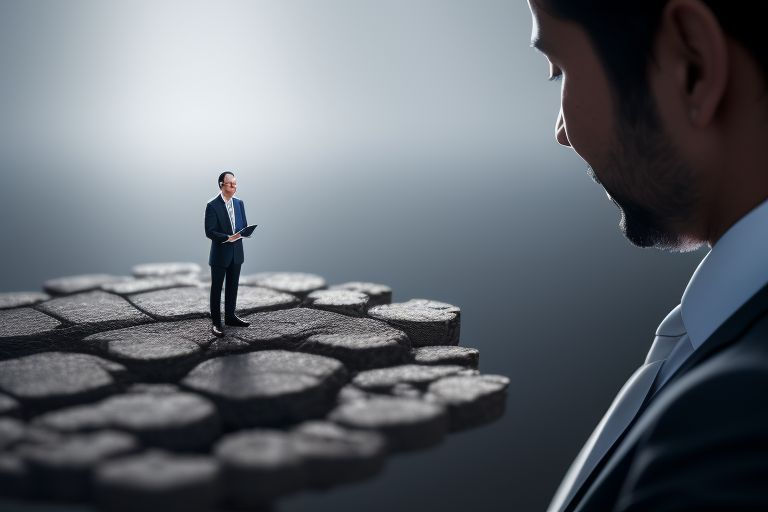
In today’s cybersecurity landscape, artificial intelligence (AI) and machine learning (ML) have evolved from trendy buzzwords into essential components of modern security. These technologies are reshaping cybersecurity by automating complex tasks, enhancing threat detection, and allowing proactive risk management at an unprecedented scale. Security leaders now have powerful tools to defend against an ever-expanding range of threats, and the impact on security operations is transformative. In this article, we’ll explore how AI and ML are enabling organisations to predict, detect, and respond to threats more effectively—and how these technologies are shifting security strategies from reactive to proactive.
AI & ML: New Allies in Security Leadership
AI-Powered Security Operations
AI and ML aren't just tools for increasing speed; they’re fundamentally changing the way security leaders tackle cyber defence. Through real-time insights and predictive capabilities, AI-driven tools help security teams see threats from new angles, empowering them to manage the rising tide of sophisticated attacks more effectively.
One of the most impactful areas for AI is in automated threat detection and response. In Security Information and Event Management (SIEM) systems, for instance, AI has revolutionised the way security data is processed. By applying ML to large datasets, these systems can identify potential threats faster and with greater accuracy than any human team. Traditional SIEMs would rely on predefined rules, but AI-driven SIEM platforms go beyond by continuously learning from patterns, adapting to new threats, and filtering out noise so that only the highest-priority alerts are escalated.
AI’s capabilities also extend to anomaly detection. By learning the baseline behaviour for users and systems, ML models can recognise even subtle deviations from expected patterns, signalling possible intrusions. For example, unauthorised access attempts or unusual data transfers are detected as anomalies, often before they manifest as full-fledged attacks. This proactive anomaly detection can mean the difference between stopping a threat early and dealing with a larger incident later.
User and entity behaviour analytics (UEBA) adds another layer to AI's role in security, analysing users’ behaviours over time to detect unusual activity. Instead of depending solely on static rules, UEBA learns each user’s routine, so any deviation—like accessing high-value assets they don't usually interact with or logging in during odd hours—raises an alert. By understanding user behaviour more comprehensively, AI-driven UEBA systems provide a dynamic approach to identifying threats that traditional methods might miss.
After AI detects a potential threat, it can assist with incident triage, classifying incidents by severity and context. For example, AI can analyse whether an attack involves brute force attempts, data exfiltration, or malware, then suggest relevant response actions. This prioritisation helps security teams address critical incidents swiftly and accurately without being overwhelmed by the sheer volume of alerts in modern networks.
AI’s predictive power is another key benefit, enabling security teams to model potential future attacks. By examining historical data, AI can anticipate the most likely attack methods and targets, allowing organisations to pre-emptively bolster defences. AI-based predictive threat modelling can also inform resource allocation, helping security leaders stay a step ahead of attackers.
AI supports digital forensics by accelerating investigations. AI-driven forensic tools can analyse logs, network traffic, and event data, pinpointing the origin and scope of an attack more quickly than manual methods. Not only is this faster, but it also reduces the risk of human error, allowing security teams to respond to incidents with precision and confidence.
Security Automation in Action
AI-driven automation is transforming security workflows by reducing manual tasks and enabling teams to focus on high-level challenges. From phishing detection to vulnerability management, automation powered by AI has become crucial to efficient security operations.
Phishing, one of the most common forms of cyberattack, can now be mitigated through AI-powered email security. These platforms analyse incoming emails for indicators like suspicious language, unusual sender behaviour, or embedded links to malicious sites. By identifying and flagging these emails before they reach end-users, AI reduces the likelihood of successful phishing attempts and keeps sensitive information secure.
AI also plays a major role in vulnerability management. Traditionally, security teams would manually review a vast number of vulnerabilities, determining which to address first. Now, AI-driven tools can analyse vulnerabilities based on criteria like exploitability, potential impact, and asset criticality, helping teams prioritise their efforts where they’ll have the most impact.
Automating access review is another critical use case for AI. By analysing user roles, permissions, and behaviour, AI-powered systems can quickly spot over-privileged accounts, orphaned accounts, and other potential risks. AI enables security teams to streamline access review processes, reducing both the time and risk involved in managing user permissions.
In configuration management, AI continuously monitors systems for misconfigurations—a leading cause of security breaches. AI-driven tools scan for deviations from security standards and can even make automated adjustments to ensure configurations remain compliant and secure. This constant oversight reduces the window of time between detecting and remediating misconfigurations, strengthening overall security posture.
When it comes to compliance monitoring, AI helps organisations meet regulatory standards by automatically tracking compliance across systems, networks, and data. Automated compliance checks can identify any gaps, generate reports, and initiate corrective actions to ensure ongoing adherence to requirements like GDPR, HIPAA, or PCI-DSS.
Strengthening Security Leadership with AI Governance and Risk Management
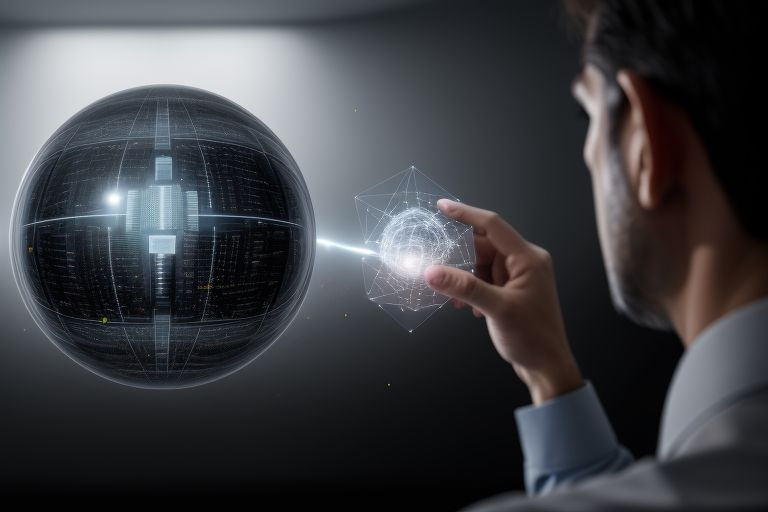
AI offers extraordinary capabilities for security, but its implementation comes with unique risks, from biases in algorithms to adversarial manipulation. Security leaders must establish governance and risk management frameworks to ensure that AI-driven tools are secure, fair, and compliant.
Building an AI Security Framework
A robust AI security framework starts with securing the models themselves. AI models—used for everything from threat detection to incident response—must be safeguarded from unauthorised access and tampering. Measures like version control, encryption, and access management are essential to maintain model integrity. Model validation before deployment is another critical step to ensure AI behaves as expected in live environments.
The data used to train AI models also requires protection. Since AI learns from vast datasets, the quality and security of this data are paramount. Organisations must secure sensitive data during collection, storage, and processing stages to prevent corruption, theft, or manipulation, all while staying compliant with privacy laws like GDPR.
Another critical aspect of AI governance is monitoring and correcting algorithm bias. Bias in AI models, often stemming from unbalanced training data, can result in unfair decisions that impact critical areas of security operations. Organisations need processes to identify and mitigate bias, ensuring that AI models make equitable and reliable decisions.
AI models require ongoing monitoring, not just for performance but also to detect security vulnerabilities. As AI models learn and adapt, they can experience “model drift,” where performance deteriorates over time. By continuously tracking model accuracy and decision-making, security leaders can identify any issues early and address them proactively.
Ethical principles must also guide AI’s use in security. Clear ethical guidelines help ensure that AI-driven security decisions respect privacy, transparency, and fairness. Security leaders should incorporate these principles into their AI strategies, particularly where AI impacts user data or sensitive decisions.
As regulatory environments evolve, organisations must integrate compliance into their AI governance. By incorporating data protection laws and industry standards, security leaders can keep their AI systems legally sound and ensure they operate in a way that respects users' privacy.
Mitigating AI-Specific Risks
With great power comes great responsibility, and AI introduces new security risks that organisations must address. For example, model poisoning, where adversaries manipulate training data to disrupt AI behaviour, requires a secure training process and data validation. AI systems also need protection from adversarial attacks, where attackers use specially crafted inputs to trick AI models. Techniques like adversarial training can help AI models recognise and defend against these attacks.
Regular AI system audits are essential to maintain transparency, accountability, and compliance. Audits should assess AI performance, security, and adherence to ethical guidelines, identifying areas for improvement and ensuring that AI systems are operating as intended.
Bias monitoring is also crucial. As data and models evolve, new biases can emerge, affecting outcomes in subtle but impactful ways. By continuously monitoring and correcting for bias, security leaders can ensure that AI remains fair and effective over time.
Performance degradation, or “model drift,” occurs as AI models face changing environments. Detecting degradation early allows teams to retrain models, adjust parameters, or deploy new models, ensuring AI’s effectiveness doesn’t wane over time. Finally, AI security testing should be regular and rigorous, covering traditional tests and new vulnerabilities specific to AI, like adversarial robustness and resilience.
Balancing Innovation with Responsible AI Use
AI has fundamentally changed cybersecurity, offering unparalleled capabilities to detect, predict, and respond to threats. But as security leaders adopt these tools, they must balance innovation with governance, ensuring that AI remains a force for good. By establishing a strong AI security framework and implementing risk mitigation strategies, organisations can harness AI’s potential while safeguarding against its unique risks. When managed responsibly, AI will continue to transform security operations, offering organisations new strength and resilience in the face of evolving cyber threats.
At Spartans Security, we are committed to helping organisations navigate this new era of AI-driven security. Our expert team is ready to support you with tailored AI security solutions, risk assessments, and ongoing guidance to ensure your AI systems remain safe, effective, and ethically sound. Contact us today to learn how we can help you build a secure AI framework that’s built for the future.
Comments